Machine Learning Is Effective in Evaluating Chronic Kidney Disease Prognosis, According to a New Study.
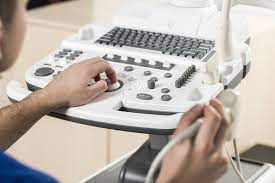
When compared to the Kidney Failure Risk Equation, three machine learning models showed equal prediction and better sensitivity in the prognosis of chronic kidney disease (CKD).
In three models: logistic regression, nave Bayes, and random forest, a study published in Scientific Reports demonstrated that machine learning was equivalent to the Kidney Failure Risk Equation (KFRE) in estimating the prognosis of chronic kidney disease (CKD).
This study used data from a longitudinal cohort that had previously been engaged in an observational study. This study covered patients aged 18 and up who had had stable renal function for at least three months. A history of renal replacement therapy, any other physically fragile condition, or any prior cancer were all considered exclusion criteria. Between April 2006 and March 2008, patients were recruited.
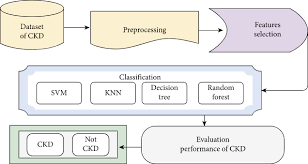
Age, gender, education level, marital status, and insurance status were all gathered as demographic data. Smoking status, alcohol consumption history, and the presence of diabetes, cardiovascular disease, and hypertension were all part of the medical history.
The study included 748 patients, with an average (SD) follow-up of 6.3 (2.3) years. At baseline, the majority of patients had stage 2 (24.5%) or 3 (47.1%) CKD, with end-stage renal disease (ESKD) detected in 9.4%, all of whom received kidney replacement therapy.
When missing data were replaced with imputed values, there were no significant differences between the imputed datasets and the original dataset. The random forest algorithm, which had overlap with the other three models, had the best overall performance.
The logistic regression model had the highest mean sensitivity (0.79; 95 percent confidence interval, 0.73-0.85), the Nave Bayes model had the highest mean accuracy (0.86; 95 percent confidence interval, 0.85-0.87) and specificity (0.87; 95 percent confidence interval, 0.86-0.89), and the random forest model had the highest mean area under curve (AUC) score (0.81; 95 percent confidence interval, 0.78-0.83) of the three models.
The AUC score of the KFRE model was comparable, and it also had the highest mean accuracy (0.90; 95 percent CI, 0.90-0.91), specificity (0.95; 95 percent CI, 0.45-0.55), and precision (0.95; 95 percent CI, 0.45-0.55). (0.50; 95 percent CI, 0.45-0.55). The KFRE model, on the other hand, has the lowest mean sensitivity (0.47; 95 percent CI, 0.42-0.52).
There were some drawbacks to this research. The cohort had fewer than 1000 participants, and ESKD was only found in a tiny percentage of them, which could have influenced the models’ performance. Furthermore, because of a paucity of urine testing when the cohort was constructed, the study solely looked at ESKD prognosis without pee.
The researchers concluded that machine learning could effectively evaluate the prognosis of CKD, with logistic regression, naive Bayes, and random forest all having equivalent predictability to KFRE. The sensitivity scores of the machine learning models were also higher, which could aid with future patient assessments.
Find the best urologist in Quetta
Click here for more information.
This is all about Evaluating Chronic Kidney Disease
Author: Muhammad Asim